|
|
Stochastic transcription | Genomics | Next Generation Sequencing | Bioinformatics tools| Overview for non-scientists
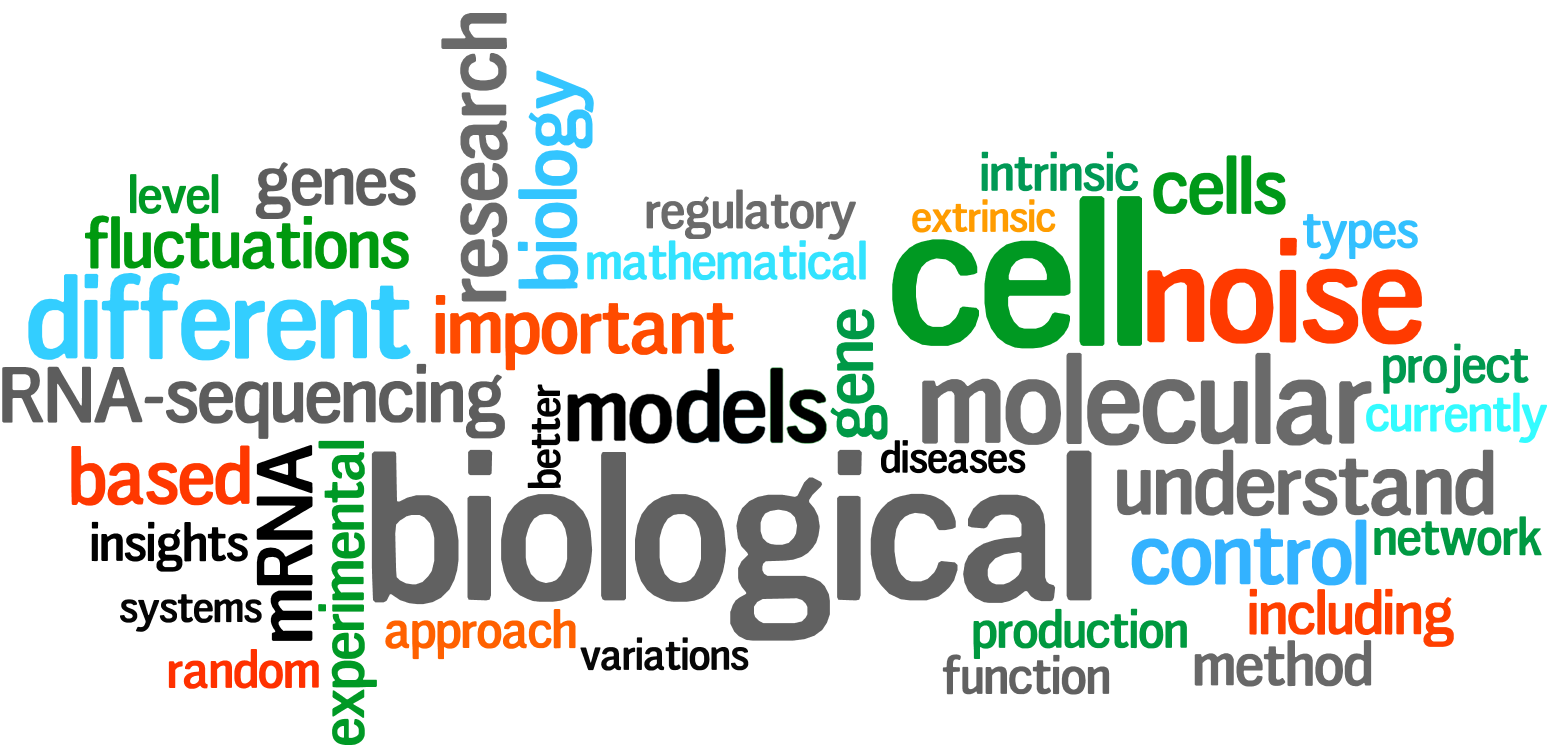
Research - stochastic transcription
Transcription in mammalian cells is a strongly fluctuating process and produces mRNAs in 'bursts', which gives rise to broad distributions of mRNAs in individual cells.
Several factors contributing to the irregular dynamics of transcription have been identified and
include the probabilistic nature of reactions due to low molecule numbers, the cell cycle, cell size fluctuations, and others.
However, most of these factors can only partially account for the observed 'transcriptional noise',
and some possible contributors have not been explored yet.
We are using various techniques such as single-molecule RNA-FISH (see image below), next generation sequencing, genomics, and bioinformatics to investigate this subject from different angles.
References:
Cavallaro M et al. Polymerase recycling contributes to transcriptional noise.
Hebenstreit D et al. RNA sequencing reveals two major classes of gene expression levels in
metazoan cells.
Hebenstreit D et al. Duel of the fates: the role of transcriptional circuits and noise in CD4+ cells.
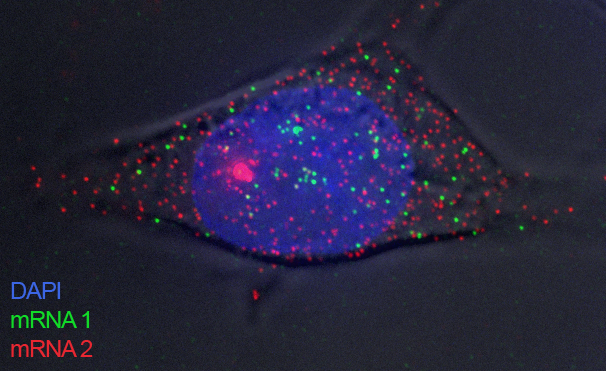
Single-molecule RNA-FISH
Genomics
The availability of many genome sequences, together with the development of next generation sequencing based assays, has produced a wealth of data that
permits genome-wide analyses; comparing thousands of genes or other features can reveal trends that suggest mechanisms (see image below; genes separate into two groups based on expression level and H3K9/14ac epigenetic marks).
Using techniques such as RNA-seq, ChIP-seq, and PRO-seq, we generate genome-wide data that helps identifying mechanisms involved in stochastic transcription.
References:
Hebenstreit D et al. RNA sequencing reveals two major classes of gene expression levels in
metazoan cells.
Hebenstreit D et al. Analysis and simulation of gene expression profiles
in pure and mixed cell populations.
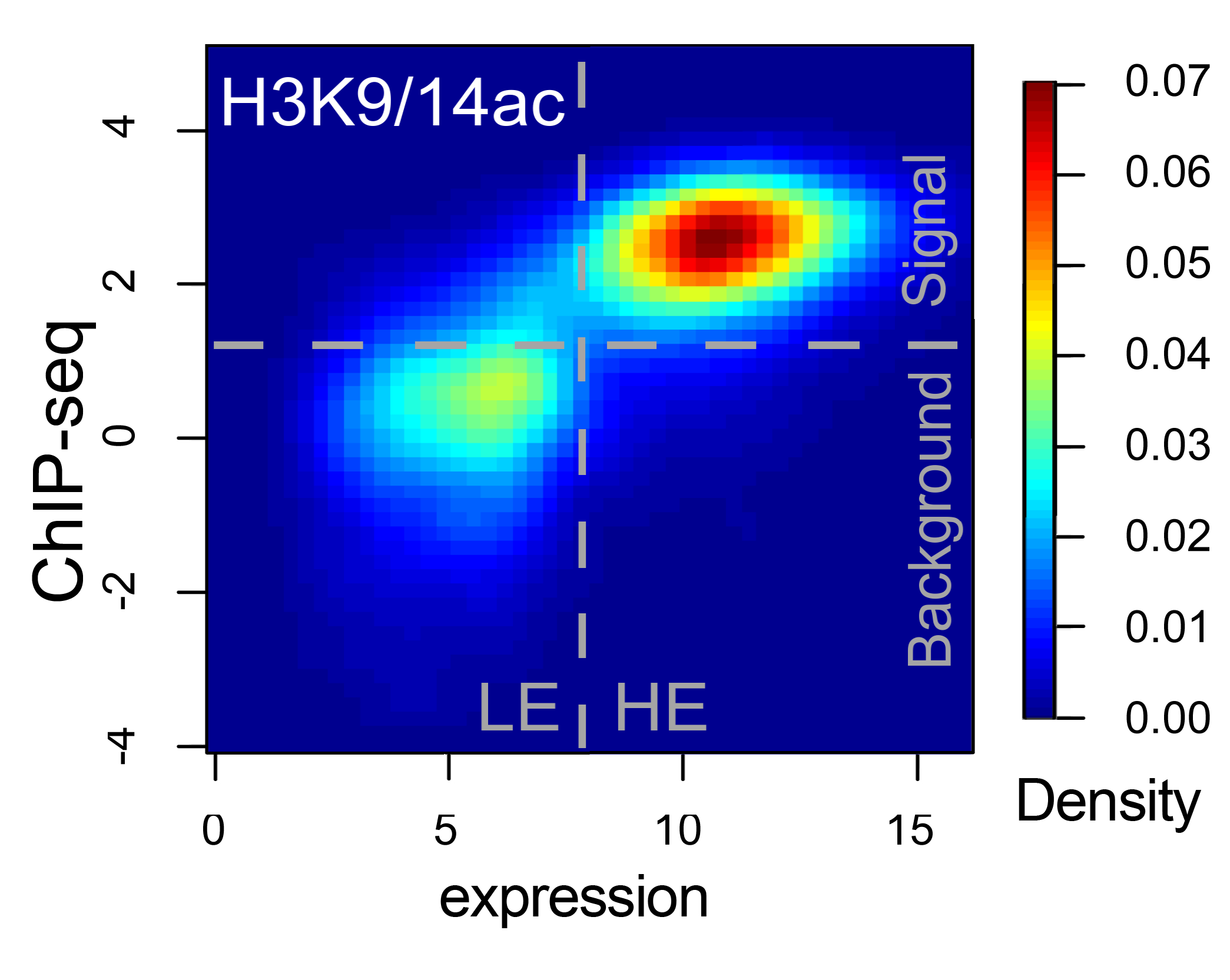
From [1]. Colour indicates density of genes.
Next generation sequencing
As part of our efforts to measure biological quantities with high precision, we also want to experimentally and computationally improve methods, in particular next generation sequencing based assays.
A technique such as single-cell RNA-seq is a powerful approach to analyse stochastic transcription, as, in principal, it outputs absolute mRNA numbers for all genes for single cells.
However, it suffers from low sensitivity and precision; >90% of mRNAs in a cell remain undetected and quantitation of mRNA numbers is subject to inherent biases in experimental procedures.
We have recently developed a way to visualize (see image below), analyze, and correct global biases in RNA-seq data using Bayesian statistics.
References:
Hebenstreit D. Methods, Challenges and Potentials of Single Cell RNA-seq
Archer N et al. Modeling Enzyme Processivity Reveals that RNA-Seq Libraries Are Biased in Characteristic and Correctable Ways
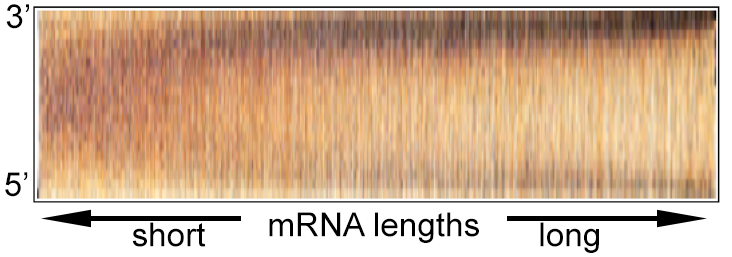
Adapted from [2].
mRNAs detected by RNA-seq are lined up at 5' and 3' ends and ordered from short to long. Colour indicates density of sequencing reads and shows an ogival global bias.
We have developed some of our analysis strategies into generally usable tools.
EpiChIP software allows quantifying epigenetic marks relative to genomic coordinates based on their global distribution:
epichip.sourceforge.net
LiBiNorm software allows global bias analysis and removal for RNA-seq data:
www2.warwick.ac.uk/fac/sci/lifesci/research/libinorm/
References:
Hebenstreit D et al. Gene-by-gene quantification of epigenetic modication levels.
Overview for non-scientists
Cells are complex machines functioning on a molecular scale. They are often compared to electronic devices: microscopic units that process information.
Electronics might be regarded as fixed infrastructures that provide switchable channels for electric current. The devices are designed robustly enough to permit two vastly different levels of current, allowing the familiar approach of binary logics to design circuits and make them modular.
Things are very different in biological cells: nothing is ‘fixed’, everything is in flux.
Individual components of cells undergo state transitions but also strongly vary in numbers.
Individual cells will have vastly different numbers of a specific mRNA, even if the cells are genetically identical and are kept under identical conditions, for instance. Instead of a fixed infrastructure, cells are thus better regarded as highly complex joint probability distributions over their components;
there is a large degree of stochastic variation.
A better understanding of how cells work and how they can be manipulated is therefore a
formidable challenge.
We want to advance the field by employing an interdisciplinary approach based on precise measurements, data at single-cell, single-molecule and genome-wide resolution, and theoretical analysis.
|
|
|